Introduction
Fans of science fiction books or movies have all experienced a sequence when someone who has not yet committed a crime is arrested because AI predicts they will in the coming hours or days. Can this impressive outcome be experienced today for networks?
Networks are complex, consisting of interconnected devices with dynamic interactions at management, control, and data planes, with many external parameters (traffic usage, software bugs, human errors, config changes, environmental conditions, physical contacts etc.). Moreover, network data for operations is prolific and extremely noisy. It is however possible to use unsupervised techniques to automatically detect without human effort in the haystack of network data insightful pattern changes that can help network operators to anticipate a failure from happening well in advance to avoid the outage.
This blog will discuss an example experienced in production networks with Augtera network AI.
Optical Failure
Link up and down are not rare in a network. Some are planned and intentional (e.g., maintenance) others are due to are unexpected failures (e.g., fiber cut during road construction).
There is a third category related to gradual degradation of the transponder or optical transport. This third category can be captured by optical metrics on the router interface using SNMP polling, or telemetry streaming, as this is the case in following example.
The following Anomaly showing up on customer network topology displays two pieces of information related to the laser received optical power
metric of one core physical interface:
- The link went down around midnight (red anomaly on the right)
- The first anomaly detecting abnormal pattern change on that metric started about 12 hours before (in red on the left of the graph)
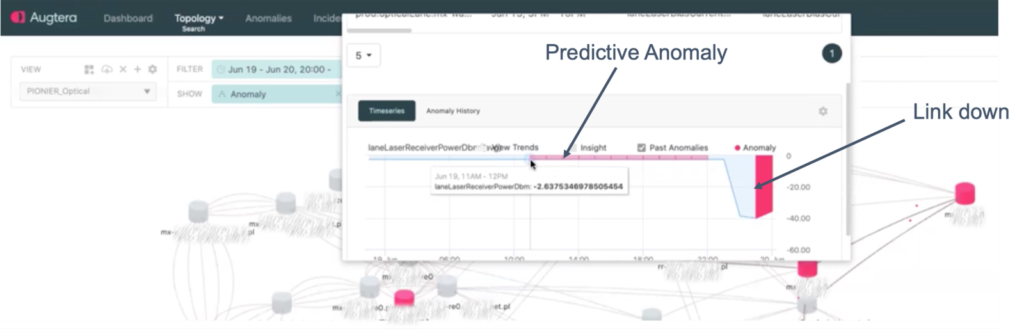
Figure 1- Optical ML-based anomaly on laser received optical power
metric (Dbm)
The pattern change detection resulting from Augtera purpose-built ML algorithm is difficult to catch with the human eye. Inspecting the data of the anomaly is possible in one click: it provides more granularity on the graph, and now we can get a sense of the subtle change in the signal.
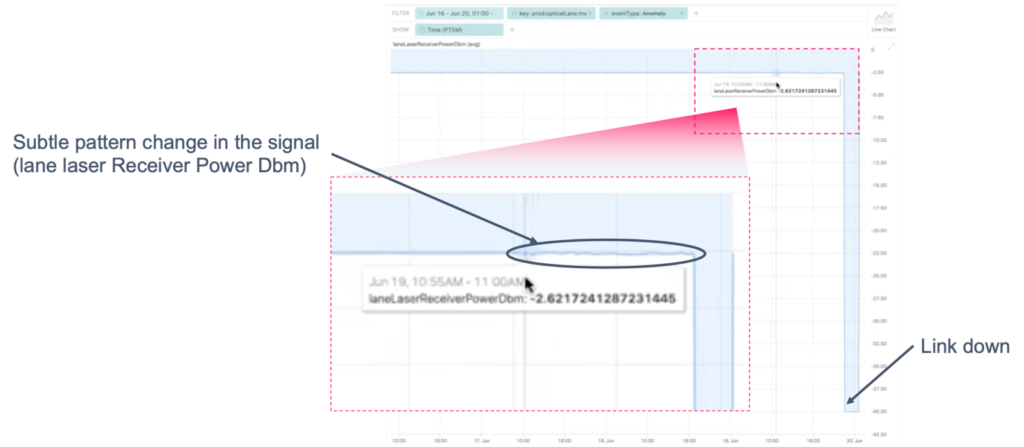
Figure 2- Detail visualization of the data related to the lane laser receiver power anomaly
As a result, Augtera Network AI detected an anomaly on optical power with a pattern change happening 12 hours before the incident.
This impressive analysis from machine learning is not likely from a Network Operations team, as the level of perturbation looks very small, and one may think that this was just a coincidence, i.e., a false positive. However, the reality is that from a mathematical standpoint, looking at historical data and behaviors on similar components in this network, the pattern change that happened is significant enough to justify the anomaly.
Confirming the Detection
This incident offered us a second anomaly that confirms the above assessment. At the same time, and on the same interface lane laser object, we had a series of ML anomalies related to the laser bias power setting current metric. The laser bias provides direct modulation of laser diodes and modulates currents. This time the pattern change is visually impressive and not debatable, starting about 12 hours before the failure.
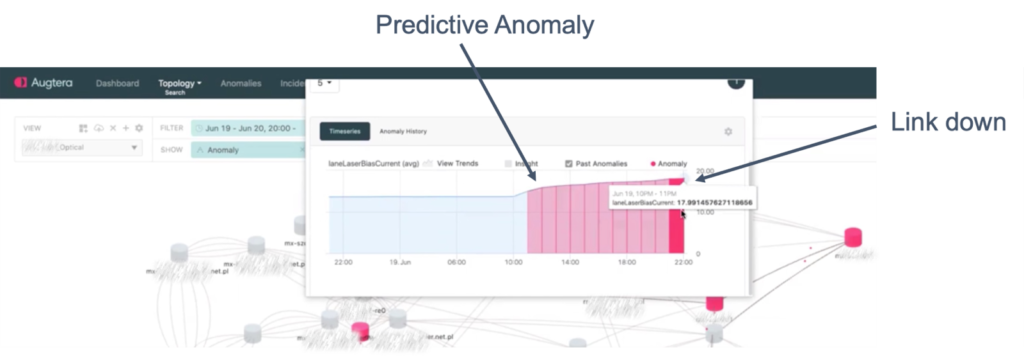
Figure 3- Optical ML-based anomaly on lane laser bias current (mA)
A quick look at the graph of laser bias current metric following the same one-click workflow allows the network operator to further confirm visually the misbehavior of that interface before the incident.
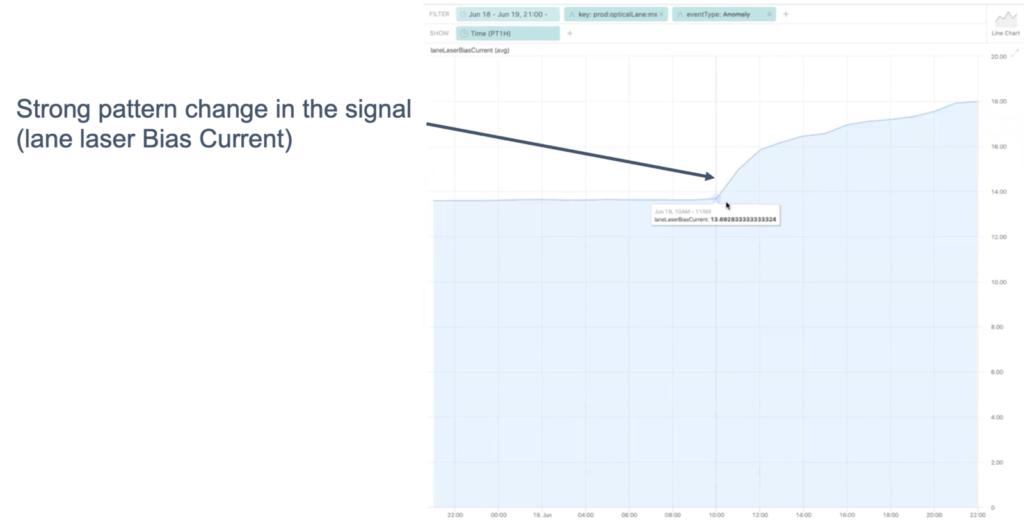
Figure 4- Detail visualization of the data related to the laser bias current anomaly
Conclusion
The conclusion of this incident and Augtera Network AI anomalies are two-fold:
- Some optical failures can be presaged with automated purpose-built ML technology, able to detect anomalies on strong or subtle pattern changes while eliminating false positives. Both optical power and bias current metrics have proven to be effective in production networks to detect optics gradual degradation before failure.
- The Network operator can now detect future optical failures to prevent the outage. The operator can fix the issue during a maintenance window, potentially during working hours if desired, and without service interruption
The operator was trialing Augtera Network AI software and did not take action on this anomaly. However the operator started to do so for future anomalies as they developed confidence in the ML anomalies as a result of this scenario.
To schedule a 30-minute discussion with an engineer on how Augtera Network AI can help with your network challenges please click the contact us link Thanks for reading our blog.