Introduction to ECN and PFC in Data Center Fabric
In the dynamic realm of data center operations, especially those focused on training large and distributed AI models, network congestion can significantly impact efficiency and performance. Understanding the role of Explicit Congestion Notification (ECN) and Priority Flow Control (PFC) is crucial in this context. These two mechanisms work in tandem to manage congestion in data center fabrics, ensuring smooth data flow and optimal network utilization. Let’s start with some basic introduction for those not sufficiently familiar.
Explicit Congestion Notification (ECN)
What is ECN?
ECN is a network mechanism used to signal congestion in a network without dropping packets. It is used in IP networks to manage data traffic effectively. When a network device (like a router or switch) experiences congestion (i.e., when its buffer is getting full), it marks the packets, signaling that there is congestion on the path.
How ECN Works
Packets in an IP network have an ECN field. When a device in the network path is experiencing congestion, it sets a value in the ECN field of the packet. This marked packet, when it reaches its destination, informs the receiver that there has been congestion along the route. The receiver can set the ECN-Echo (ECE) flag in the TCP header of the acknowledgment packet sent back to the sender or signal it at application level for UDP transport. The sender, upon receiving this notification, reduces its transmission rate. This is known as reducing the congestion window. The process helps in preventing packet loss and avoids the need for retransmissions, which can be costly in terms of bandwidth and time.
Priority Flow Control (PFC)
What is PFC?
PFC is a mechanism used in Ethernet networks to prevent packet loss during congestion, specifically in scenarios where time-sensitive data is being transferred. PFC works by temporarily pausing transmission of data on a congested network path.
How PFC Works
In a congested state, a device (like a switch) sends a PFC frame to its connected device (like another switch or a server), instructing it to stop sending data on specific priority lanes (Ethernet has multiple priority lanes for different types of traffic). The pause frame specifies the duration for which the sender should stop sending packets. This pause allows the congested device to clear its buffer and avoid dropping packets. Once the buffer is cleared, and the pause time expires, the sender resumes sending data.
Interaction of ECN and PFC in Data Center Fabrics
Coordinated Approach
In a data center environment, especially in Ethernet fabrics used for AI model training, both ECN and PFC play pivotal roles in managing congestion. ECN is more about providing feedback for congestion control over a longer duration, allowing for dynamic adjustment of data flow rates. PFC, on the other hand, provides an immediate response to congestion, pausing specific traffic types to prevent packet loss in real-time.
Synergy in High-Performance Environments
The synergy between ECN and PFC is critical in high-performance computing environments like those used for AI model training. ECN helps in maintaining overall network efficiency by adjusting the flow of traffic based on congestion feedback. PFC ensures that critical, time- sensitive data is not lost during temporary congestion spikes.
Importance in AI Model Training
During AI model training, large volumes of data are transferred between GPUs. Congestion in the network can lead to delays and packet loss, impacting the training process. By using ECN and PFC in tandem, the Ethernet fabric can manage traffic flows, prevent packet loss, and maintain high throughput, which is essential for the timely completion of AI model training tasks.
Key Metrics: ECN and PFC Monitoring
Augtera’s Network AI platform excels in monitoring specific metrics crucial for congestion management, particularly ECN packets (both sent and received) and PFC Pause frames (sent and received), along with a wider range of network metrics. These metrics offer a granular view of the network’s health and are essential in preempting and managing congestion scenarios, at second level precision.
The Impact of Congestion in AI Model Training
Congestion in a data center fabric, particularly one utilized for training distributed AI models, can have far-reaching effects. It not only slows down the training process but can also lead to inefficient resource utilization and increased operational costs. Therefore, identifying congestion early is vital to maintain the efficiency of these processes.
Early Indicators: Deciphering ECN and PFC Anomalies
Augtera’s Network AI platform highlights the importance of recognizing ECN and PFC anomalies as early indicators of congestion. However, it is crucial to understand that these are symptoms rather than causes. The root cause could range from network issues, like a failing interface, to overload situations at destination servers or GPUs.
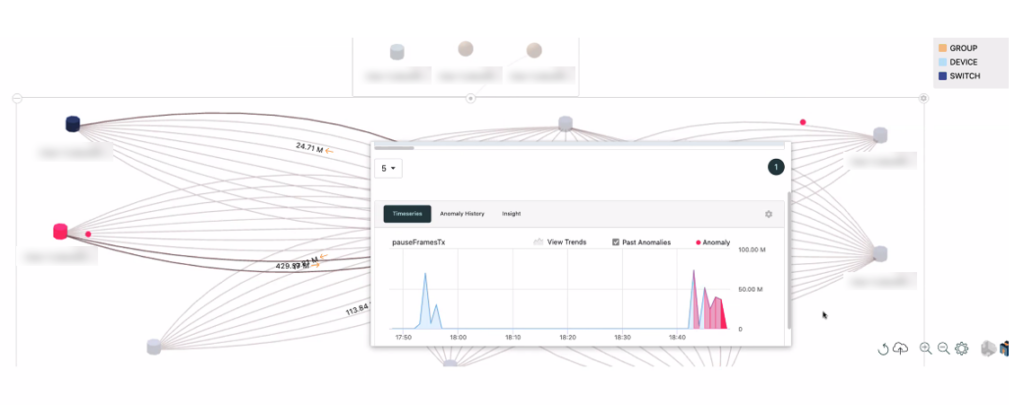
Holistic View with Augtera: Identifying the True Cause of Issues
One of the strengths of Augtera’s platform is its ability to provide a holistic view of various network events. This comprehensive perspective is crucial in pinpointing the source of a problem – be it a network, server, GPU, or application issue. Such clarity is indispensable for prompt and effective resolution.
For example, the detection of an anomaly in PFC pause frames may happen simultaneously with other events an anomalies such as an interface in the fabric going down, traffic anomaly on the remaining interfaces on the Aggregate and likely, as a consequence, the generation of PFC pause frames. It is therefore important not only to rapidly detect the congestion situation but also to identify what triggered it.
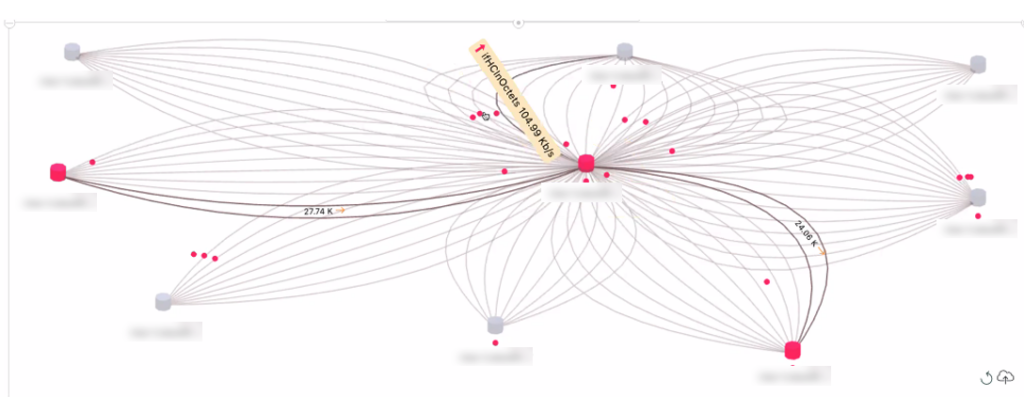
In the diagram above, multiple simultaneously detected events and anomaly are detected and displayed. The correlation algorithm will connect the events using ML based topology aware correlation and identify not only the connections between the events but also help the operator identify the root cause.
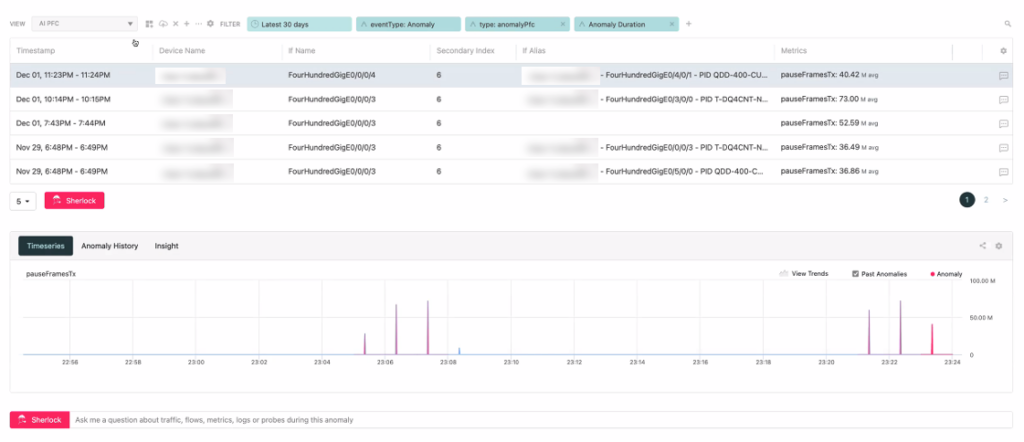
The Importance of Real-Time Monitoring and Granularity
In the fast-paced environment of AI model training, congestion situations can be transient and triggered by a myriad of factors. Augtera’s platform offers real-time monitoring with second-level granularity, a critical feature for detecting and addressing these fleeting congestion scenarios. This level of detail helps in identifying the root cause, whether it’s a hardware, software, GPU issue, or even memory errors.
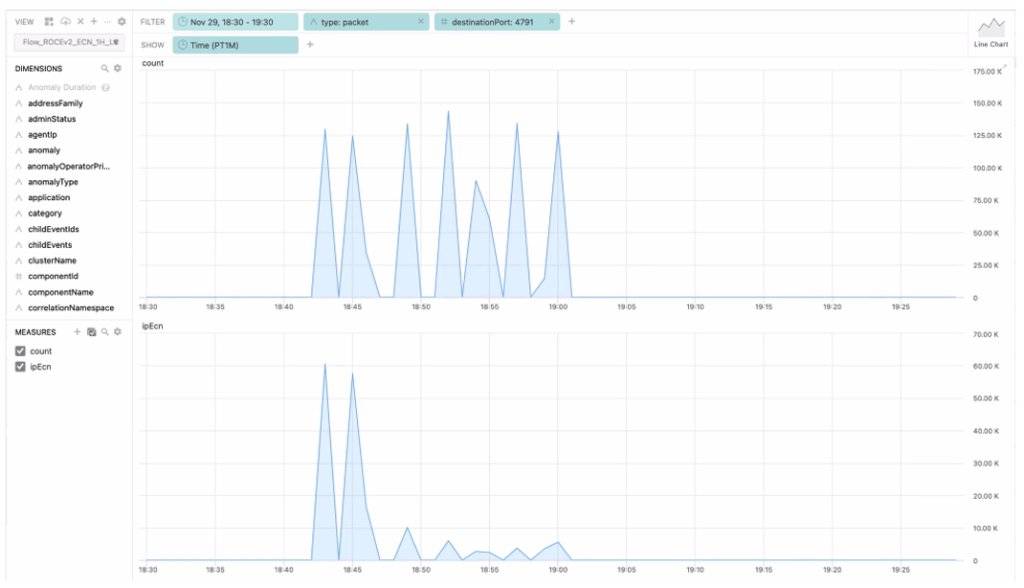
Conclusion: Augtera’s Unique Industry Solution
In summary, Augtera’s Network AI platform offers unparalleled real-time visibility into congestion states by monitoring end system reports via ECN and network reports via PFC. By correlating this data with a broad range of network, server, and GPU metrics, Augtera provides a unique AI-enabled observability solution for large-scale AI Ethernet clusters. This capability not only enhances efficiency in AI model training but also ensures the optimal performance of data center fabrics.