Contact us to arrange for a demo from one of our engineers
Introduction – IT Ops and Net Ops are not the same
IT Ops and Network AIOps are very different1. At some abstract level, all AIOps offerings, across all of IT, share some similar characteristics. This results from well-known operations challenges and from the well-known definition created by Gartner. Common themes include:
- Anomaly detection
- Performance analysis
- Correlation
- Incident task management
- Automation
However, most of the vendors Gartner includes in AIOps, are not offering deep networking solutions. AIOps was a term that arose from challenges in application and compute. The micro services approach to software architecture broke the approaches to operations tooling that had been successfully used, and a new approach was needed.
Similarly, in networking, it can be argued that new dense-topology network architectures based on fixed form-factor switching / routing, the emergence of hybrid / multi-cloud, underlays, overlays, SD-WAN / SASE and more, broke what had previously worked in network operations.
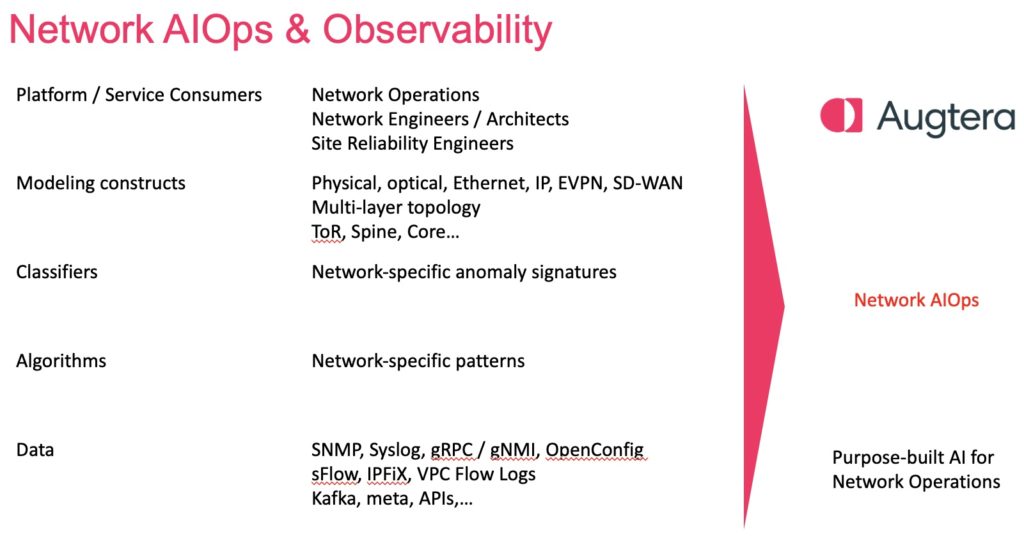
However, while some similar branches of AI/ML & data science may be applied in IT Operations and Network Operations, the fidelity depends on understanding the domain and providing data inputs that will address the use cases in each domain.
Network AIOps Speaks Networking
For some aspects of AI/ML it is plausible to claim being agnostic to a domain. For example, some platforms can correlate anything. How good are the answers though? Garbage in, garbage out.
The information needed to understand networking use cases comes from SNMP, Syslog, sFlow, IPFiX, network probes, network meta data, and more.
The problem to be solved is not just understanding network-specific protocols. It is also about understanding the constructs in the network that can be effectively analyzed and contextualized. Protocols, network constructs, network state machines, network models, network behavior, and more is required for effective observability and real-time detection, multi-layer topology-aware correlation, noise elimination, operations policy enforcement, noiseless ticket creation, auto-mitigation / remediation and more.
All the data science PhDs in the world cannot deliver a working Network AIOps solution without understanding what the data being ingested is all about.
Network AIOps Speaks Data Center
Do IT Ops solutions understand Top of Rack (ToR), Spine, Core, and other important concepts? Why does it matter? What if network operations want to limit alerts to upstream ToR links and not be notified every time a ToR downstream link has an anomaly? Network-centric constructs must be understood to support this kind of policy.
How about correlating data across multiple layers: physical, optical, Ethernet, IP, EVPN, TCP and more? If the AIOps solution does not collect the data at each layer, model it, understand the inter-relationships, and focus on the use cases in these layers, then it is not a Network AIOps solution.
Network AIOps Speaks SD-WAN
Network operations teams need to understand the inter-relationship between SD-WAN overlays and the infrastructure underlays. This again is the kind of use case that only occurs to Network AIOps.
Network AIOps Speaks TCP Flow Data
Looking for signs that network anomalies are impacting applications? One place to start might be TCP resets. Then next step might be to look for network fabric congestion. Lastly looking for the connections between each. This is the kind of use case that only occurs to Network AIOps.
Example Use Cases
Network congestion detection.
Machine learning learns normal buffer/queue patterns for every object.
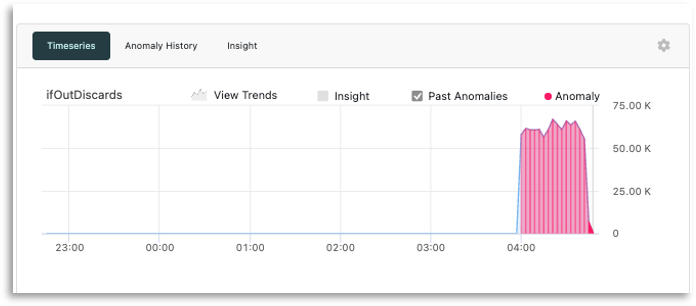
Anomalies can be overlayed on topology and/or used to generate a notification
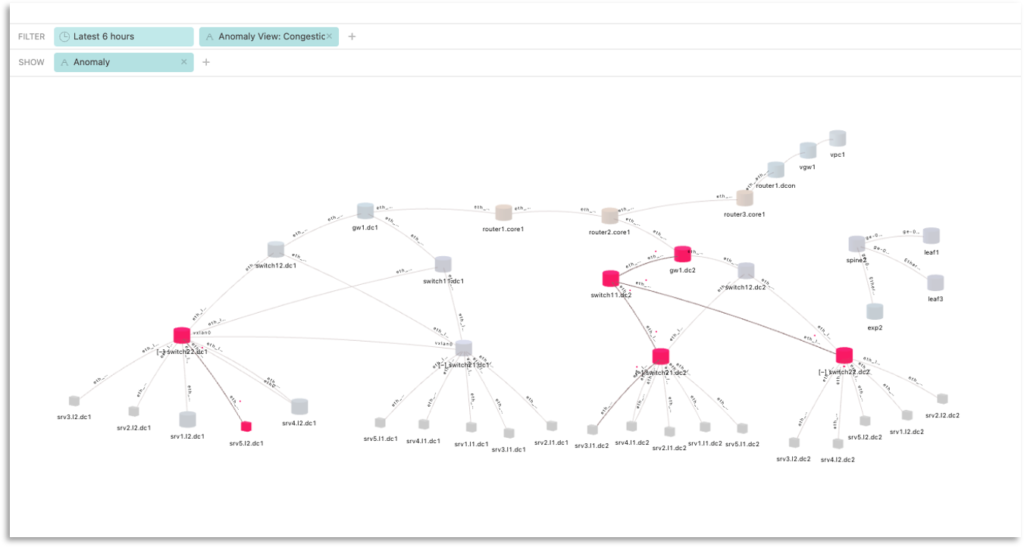
Auto Correlation of Anomalies and events
Augtera machine learning algorithm will auto correlate events (Syslog, SNMP Traps, Telemetry alarms) and machine learning anomalies.
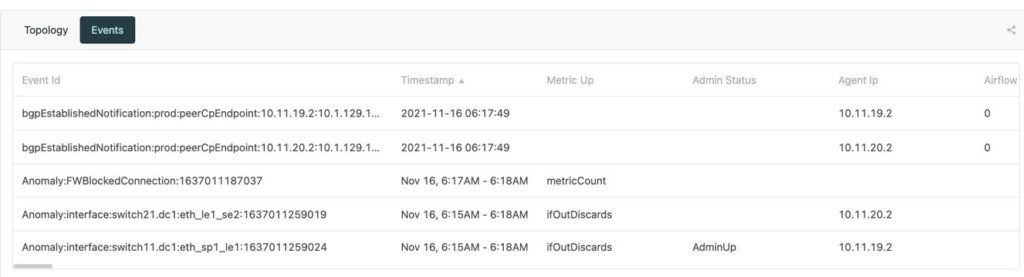
Correlated events are overlayed to impacted topology.

Application Performance
Detect and correlate application performance issues with underlying causes


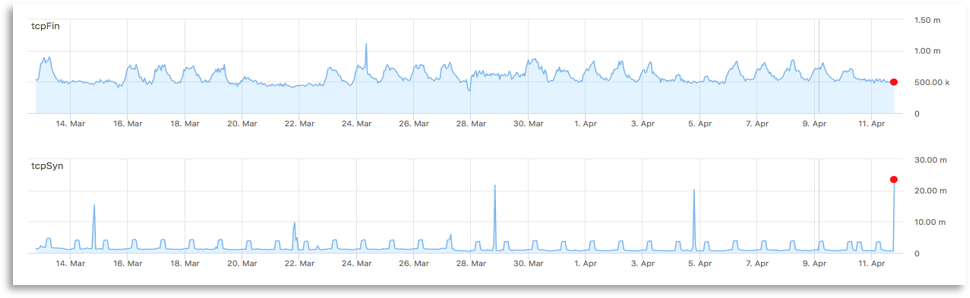
Detecting Optical Degradation / Impairments Before They Become Failures
Learn normal optical operating metrics (temp, rcv power, output power, etc.).
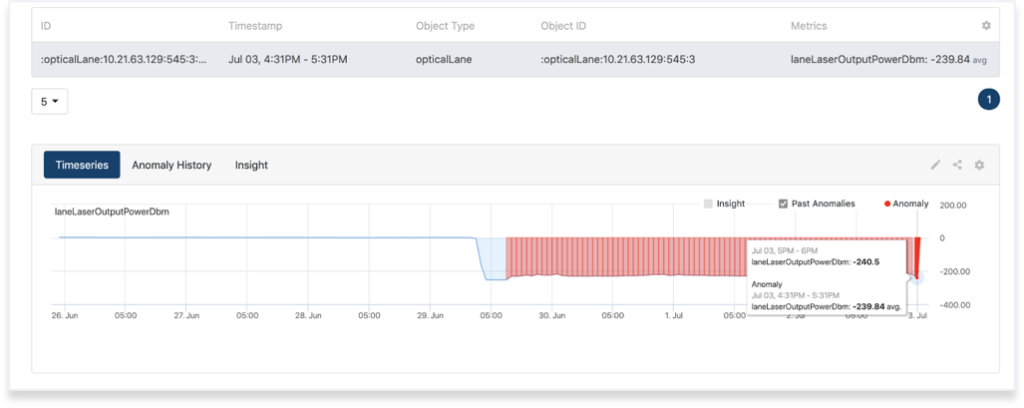
Purpose-built machine learn algorithm tuned for optical

Detecting Environmental Degradation / Impairments Before They Become Failures
Augtera retrieves environmental metrics from devices in the facility. Learns normal fluctuations and pattern per device/object. Detects abnormal changes indicating environmental issue. Applicable to temp, fan, current, voltage, etc.

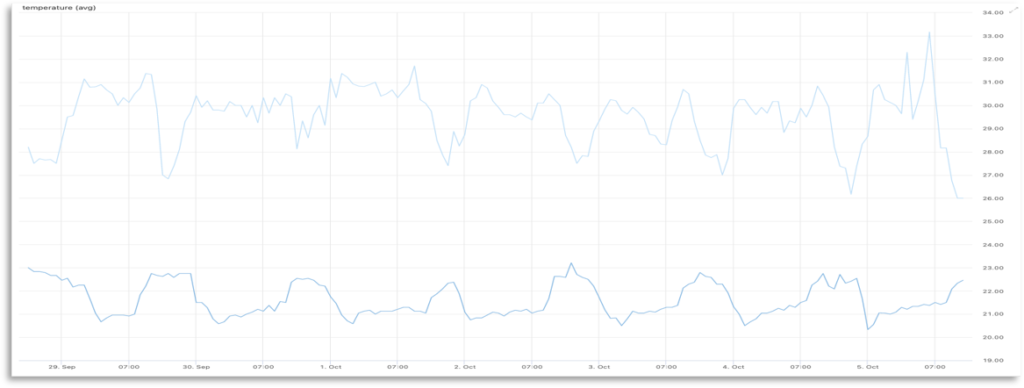
Visualize and Correlate EVPN / VxLAN Issues
Multi-Layer network model: physical, routing, overlays including VXLAN/EVPN. Anomalies mapped to VXLAN object for targeted overlay monitoring. Correlation with underlay Anomalies.
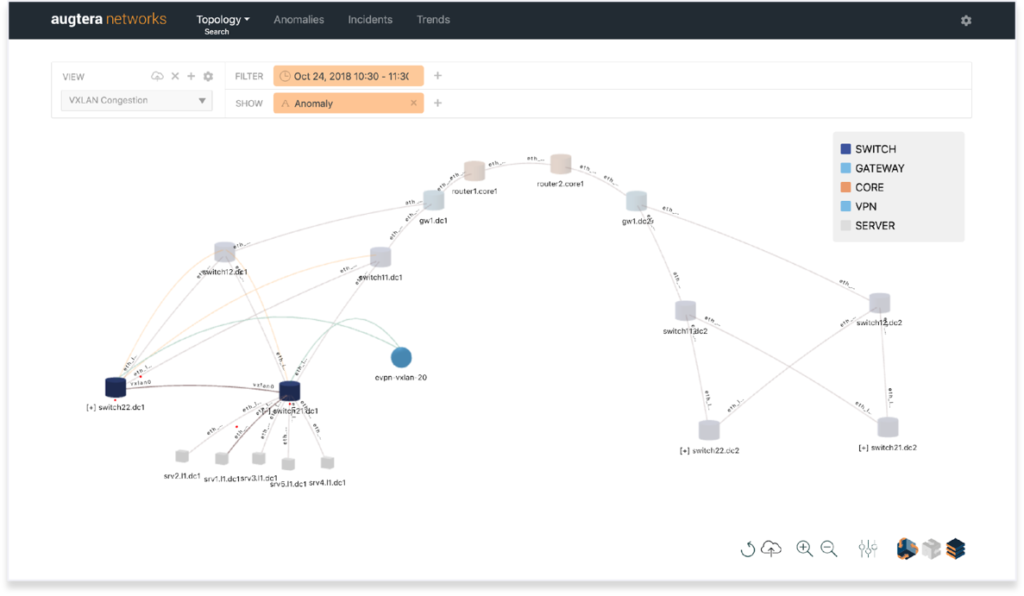
Proactive Control Plane Impairments
Machine learning builds a model for number of routes each device holds. Detect significant deviation in route counts. Can be correlated with traffic/congestion anomalies using topology.

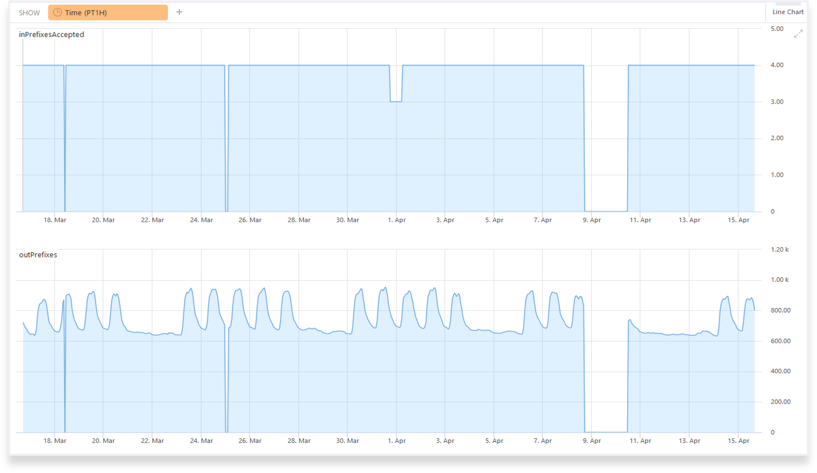
Other
- Modeling all layers of the network from the physical layer to the TCP layer and above, so effective incident root detection can be performed.
- Detection of uncommon syslog messages that are often precursors to incidents / outages.
- Understanding BGP state machines, BGP flaps, and how they impact anomaly detection and incident mitigation.
- Post maintenance verification.
- Cloud performance degradation
- Flow log insights and analysis
- More…
Conclusion
Messaging from all Observability and AIOps solutions can sound similar. The devil is in the details. What use cases are focused on? What IT roles are the solutions sold to? Do the solutions speak “network”? Do the solutions understand the stories that are told in network-only data?
Contact us to arrange for a demo from one of our engineers
Notes: (1) While we understand that Networking is part of IT, and therefore part of IT Ops in a larger sense, we are drawing the distinction between solutions focused on Networking, and solutions focused on other areas of IT.